Technolgy
Data Labeling Strategies for Industry 4.0: From Raw Data to Actionable Insights
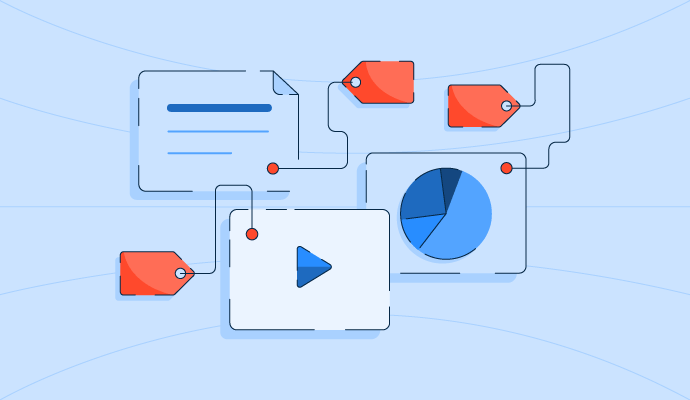
Understanding Professional Data Labeling
Professional data labeling is the process of annotating raw data, including text, images, audio, and video, to make it understandable for machine learning algorithms. This ensures that AI models can accurately classify, predict, and extract insights from the information they process.
Without proper data labeling, even the most advanced AI systems struggle to function efficiently. High-quality labeled data helps machines learn faster and improve their performance over time. Given the growing complexity of AI applications, businesses must adopt sophisticated labeling techniques to ensure accuracy and reliability.
The Role of Professional Data Labeling in Industry 4.0
Industry 4.0 integrates AI, machine learning, IoT, and automation into manufacturing, healthcare, finance, and other sectors. In this context, professional data labeling plays a pivotal role in enabling these technologies to function effectively. Some key applications include:
- Autonomous Vehicles: Labeled data is used to train AI models for detecting pedestrians, traffic signs, and road conditions.
- Healthcare AI: Labeled medical images help AI models in diagnostics, detecting anomalies, and automating patient monitoring.
- Predictive Maintenance: Labeled IoT sensor data aids in predicting equipment failures, reducing downtime, and optimizing maintenance schedules.
- Financial Fraud Detection: Properly labeled transactional data enhances fraud detection algorithms, improving security in banking and finance.
Best Practices for Professional Data Labeling
1. Define Clear Labeling Guidelines
To maintain consistency and accuracy, organizations must establish clear labeling guidelines. This includes specifying:
- Labeling criteria and classification rules
- Annotation tools and techniques
- Quality control measures
2. Choose the Right Labeling Tools and Platforms
There are various data labeling platforms available, including manual, semi-automated, and fully automated solutions. Selecting the right tool depends on the complexity of the data and the level of human oversight required.
3. Implement a Quality Assurance Process
Poorly labeled data can lead to inaccurate AI predictions. A multi-step quality assurance process, including cross-validation by multiple annotators, helps minimize errors and ensures reliability.
4. Use Active Learning for Efficiency
Active learning is a machine learning approach where the algorithm identifies uncertain data points that require human annotation. This strategy reduces redundant work and improves labeling efficiency.
5. Leverage Expert Annotators
For industries such as healthcare and finance, subject matter expertise is crucial. Leveraging domain-specific professionals for professional data labeling ensures high-quality annotations that align with industry standards.
Challenges in Professional Data Labeling and How to Overcome Them
1. Scalability Issues
As AI applications expand, so does the volume of data requiring labeling. Businesses can overcome scalability issues by implementing automation-assisted labeling and outsourcing labeling tasks to specialized firms.
2. Data Privacy and Security
Many industries handle sensitive data, making privacy a major concern. Adopting secure labeling environments, encryption methods, and compliance with data protection regulations ensures data security.
3. Ensuring Consistency in Labeling
Maintaining consistency across large datasets is challenging. Regular audits, inter-annotator agreement checks, and clear guidelines help address this issue.
4. High Costs of Professional Data Labeling
While data labeling is resource-intensive, businesses can optimize costs by prioritizing key datasets, using AI-assisted labeling, and leveraging crowdsourced annotation platforms where applicable.
The Future of Data Labeling in AI Development
As AI models become more sophisticated, the demand for high-quality labeled data will continue to rise. Emerging trends in professional data labeling include:
- AI-assisted Labeling: Combining human expertise with AI-driven annotation to enhance speed and accuracy.
- Self-Supervised Learning: Reducing reliance on manual labeling by enabling AI to learn from unlabeled data.
- Synthetic Data Generation: Creating artificially labeled data to train AI models where real-world data is scarce.
Businesses investing in advanced data labeling techniques will gain a competitive advantage in Industry 4.0 by developing more accurate, efficient, and scalable AI solutions.
Conclusion
In the digital transformation era, professional data labeling is the bridge between raw data and actionable AI-driven insights. As businesses navigate Industry 4.0, adopting best practices in data labeling will be crucial for optimizing AI performance and staying ahead in an increasingly automated world.
By implementing robust data labeling strategies, organizations can harness the full potential of AI, driving innovation and operational efficiency across various industries. Whether in healthcare, finance, or autonomous systems, high-quality labeled data will remain the foundation for AI’s success.
-
Celebrity5 months ago
Who Is Jordan Broad?: The Untold Story of Ashley Broad Husband
-
Celebrity5 months ago
Who Is Mary Ryan Ravenel?: Inside The Life Of Thomas Ravenel’s Ex-Wife
-
Celebrity5 months ago
Who Is Noelle Inguagiato?: The Untold Story Of Jesse Watters Ex-Wife
-
Celebrity5 months ago
Who Is Dolphia Parker?: Everything About Dan Blocker’s Wife